Listen to this blog
As the world enters the era of big databases, modern lifestyle produces more and more data at an unparalleled speed through apps, websites, smartphones, and smart devices. Hence, the need to store data has also expanded as the main challenge and concern among modern enterprise industries. Data science is the future of AI algorithms to overcome these challenges. It is a blend of various tools, algorithms, and machine learning life cycles with the primary goal of discovering hidden patterns from raw unstructured data.
Data science focuses on a more forward-looking, innovative approach, ensuring to evaluate historically and compare it with present data to make better decisions and future predictions on behaviour and outcomes. From healthcare and finance to cybersecurity and automobiles, data scientists significantly contribute to various breakthroughs across verticals.
Read on to learn more about the different stages in the data science lifecycle that require different skill sets, tools, and techniques.
Data science lifecycle explained
A data science lifecycle is defined as the iterative set of data science steps required to deliver a project or analysis. There are no one-size-fits that define data science projects. Hence you need to determine the one that best fits your business requirements. Each step in the lifecycle should be performed carefully. Any improper execution will affect the following step, ultimately impacting the entire process.
Data Science is key to achieving a perfect data science process here. The main aim is to build a framework and solutions to store data. Since every data science project and the team is unique, every specific data science lifecycle is different.
How many phases are there in the data analytics life cycle?
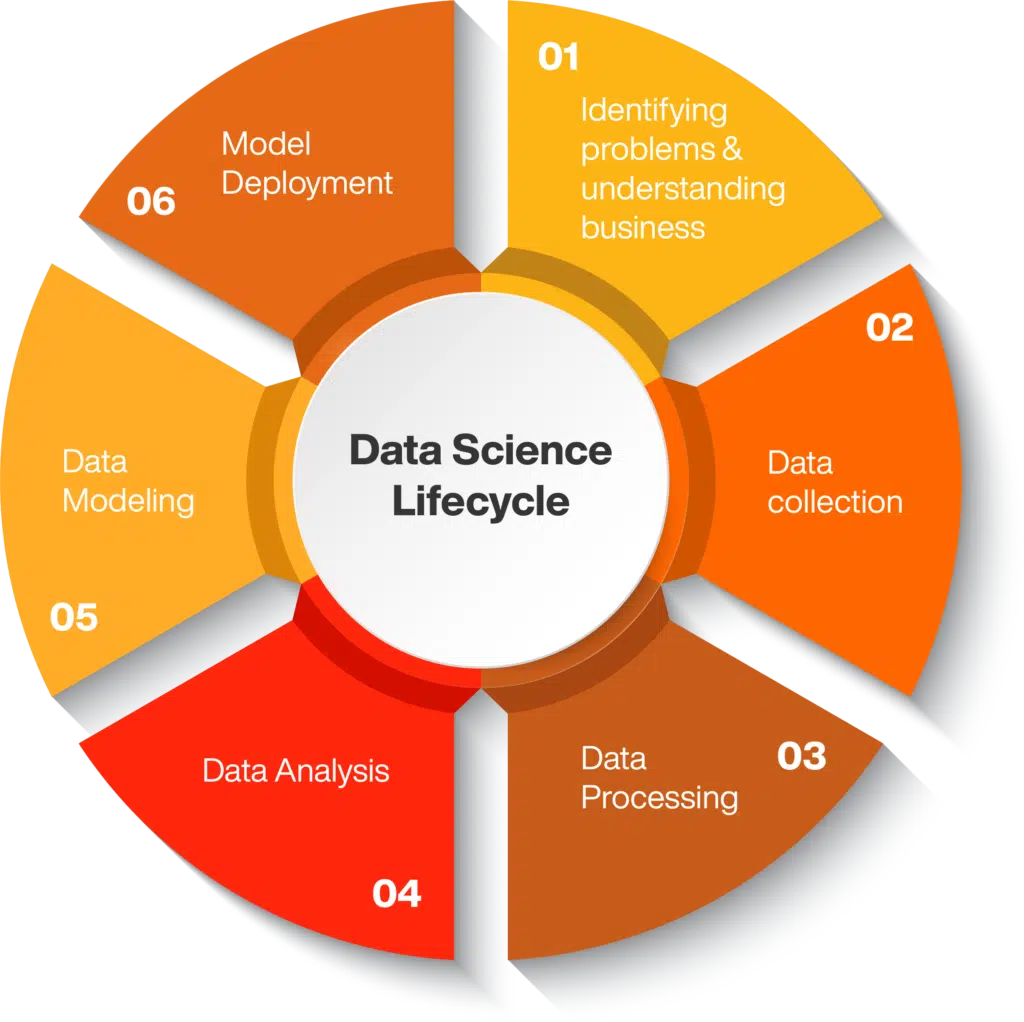
Below we have explained the different phases of data science life cycle
Phases | Description |
Identifying problems and understanding business | Discovering the answers for basic questions including requirements, priorities and budget of the project. |
Data Collection | Collecting data from relevant sources either in structured or unstructured form. |
Data processing | Processing and fine-tuning the raw data, critical for the goodness of the overall project. |
Data analysis | Capturing ideas about solutions and factors that influence the data life cycle. |
Data modelling | Preparing the appropriate model to achieve desired performance. |
Model deployment | Executing the analysed model in desired format and channel. |
- Identifying problems and understanding business
Like any other good business lifecycle, the data science lifecycle also starts with ‘why?’ Identifying problems is one of the major steps necessary in the data science process to find a clear objective around which all the following steps will be formulated. In short, it is important to understand the business objective early since it will decide the final goal of your analysis.
This phase should examine the trends of business, analyse case studies of similar analysis, and study the industry’s domain. The team will assess in-house resources, infrastructure, total time, and technology needs. Once these aspects are all identified and evaluated, they will prepare an initial hypothesis to resolve the business challenges following the current scenario. The phase should –
- Clearly state the problem that requires solutions and why it should be resolved at once
- Define the potential value of the business project
- Find risks, including ethical aspects involved in the project
- Build and communicate a highly integrated, flexible project plan
- Data collection
Data collection is the next stage in the data science lifecycle to gather raw data from relevant sources. The data captured can be either in structured or unstructured form. The methods of collecting the data might come from – logs from websites, social media data, data from online repositories, and even data streamed from online sources via APIs, web scraping or data that could be present in excel or any other source.
The person performing the task should know the difference between various data sets available and the data investment strategy of an organisation. A major challenge faced by professionals in this step is tracking where each data comes from and whether it is up-to-date. It is important to keep track of this information throughout the entire lifecycle of a data science project as it might help test hypotheses or run any other updated experiments.
- Data processing
In this phase, data scientists analyse the data collected for biases, patterns, ranges, and distribution of values. It is done to determine the sustainability of the databases and predicts their usage in regression, machine learning and deep learning algorithms. The phase also involves the introspection of different types of data, including nominal, numerical, and categorical data.
Data visualisation is also done to highlight the critical trends and patterns of data, comprehended by simple bars and line charts. Simply put, data processing might be the most time-consuming but arguably the most critical phase in the entire life cycle of data analytics. The goodness of the model depends on this data processing stage.
- Data analysis
Data Analysis or Exploratory Data Analysis is another critical step in gaining some ideas about the solution and factors affecting the data science lifecycle. There are no set guidelines for this methodology, and it has no shortcuts. The key aspect to remember here is that your input determines your output. In this section, the data prepared from the previous stage will be explored further to examine the various features and their relationships, aiding in better feature selection required for applying it to the model.
Experts use data statistics methods such as mean and median to better understand the data. In addition, they also plot data and assess its distribution patterns using histograms, spectrum analysis, and population distribution. Depending on the issues, the data will be analysed.
You may be interested in knowing more about time series analysis.
- Data modelling
Modelling Data is one of the major phases of data processes and is often mentioned as the heart of data analysis. A model should use prepared and analysed data to provide the desired output. The environment needed for executing the data model will be decided and created before meeting the specific requirements.
In this phase, the team works together to develop datasets for training and testing the model for production purposes. It also involves various tasks such as choosing the appropriate mode type and learning whether the problem is a classification, regression, or clustering problem. After analysing the model family, you must choose the algorithms to implement them. It has to be done carefully since extracting necessary insights from the prepared data is extremely important.
- Model deployment
Now, we are at the final stage of the lifecycle of data science. After a rigorous evaluation process, the model is finally prepared to be deployed in the desired format and preferred channel. Remember, there is no value for the machine learning model until it’s deployed to production. Hence machine learning models have to be recorded before the deployment process. In general, these models are integrated and coupled with products and applications.
The stage of Model deployment involves the creation of a delivery mechanism required to get the mode out in the market among the users or to another system. Machine learning models are also deployed on devices and gaining adoption and popularity in the field of computing. From simple model output in a Tableau Dashboard to a complex as scaling it to cloud in front of millions of users, this step is distinct for different projects.
RELATED ARTICLES
Who all are involved in the data science lifecycle?
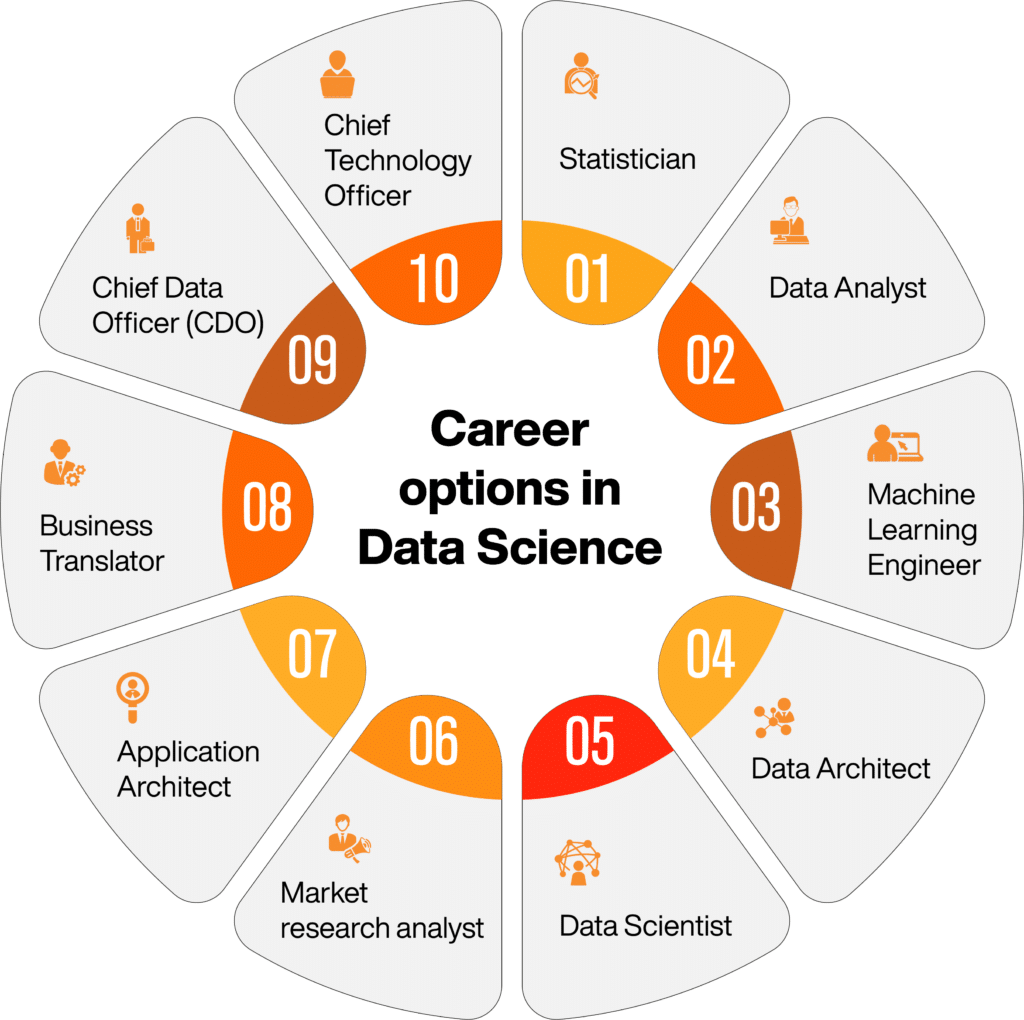
Data is being created from individual level to organisational level, gathered, and stored in substantial servers and data stores. But how are you going to get hold of this vast storage of data? It’s where the data scientist comes into play, who is an expert in the art of extracting insights and patterns from unstructured words and numbers.
Here we have listed out different job profiles of the data science team involved in the lifecycle of data science.
- Business Analyst
The role of a business analyst is to understand the business requirements in the identified domains like Banking, Healthcare, education, and more.
The responsible person can guide in planning the right solution and timeline to perform the analytics in their business analytics lifecycle. Moreover, they should also find the right target customers and analyse the effectiveness of various campaigns to create plans and smoothen business processes.
- Data Analyst
A data analyst is a data science expert who has extensive knowledge and experience in working with huge data. They can map the solution and analyse what data is required to generate the required solution. Data Analysts are required to format and clean the raw data, interpret and visualise them to perform the analysis and provide the technical summary of the same.
- Data Scientists
Data scientists take another step down in the lifecycle of data science, and their main task is to improve the quality of machine learning models. In general, they divide their work into two blocks –
- Work with the finished model of the project to assess the quality continuously and identify the areas that need to be improved.
- Gather online and offline metrics to find new architectures and signals for predictions.
- Data Engineer
Data Engineers focus on technique optimisation and data construction in a conventional manner. They are in charge of preparing data for subsequent analysis, gathered from social networks, websites, blogs, and other internal and external web sources. It will then be formed into a structured form so that it can be shared with the data analyst for further steps.
- Data Architect
The primary role of the Data Architect is to integrate, centralise, safeguard and maintain the data sources of the organisation’s life cycle. They have to work with the latest technologies to ensure the business stays on top and relevant. In addition, data architects also have a significant role in creating a blueprint for database management, organising data both at macro and micro levels.
- Machine Learning Engineer
A job profile of a machine learning engineer involves the responsibility of providing advice on which model can be applied to acquire the desired outcomes and build a solution to offer accurate output. They have to design and implement machine learning-related algorithms and applications, including prediction, and anomaly detection, to address the business challenges. They collaboratively build data pipelines, benchmark infrastructure, and A/B testing.
Conclusion
When it comes to better decision-making, data is inevitable. This is also true on an organisational level, where the majority of today’s businesses rely on data-driven decisions and strategic plans to achieve their long-term goals. Implementing a perfectly defined data science lifecycle will help you optimise and streamline business operations.
Enrol in the Master’s Programme in Data Science provided by the Manipal Academy of Higher Education (MAHE) through Online Manipal, which will help you boost your intelligence and hone your skills in data science, allowing you to emerge as a competent individual in the industry. In a nutshell, due to the accelerating rate of data sources, data science has become one of the fastest growing fields, enabling businesses to interpret data and provide actionable insights to improve outcomes.
Become future-ready with our online MBA program
View All Courses